The Critical Role of Data Strategy
No Data Strategy, No Long-Term Success: Why High-Quality Data is the Key to Business Growth and Competitive Advantage
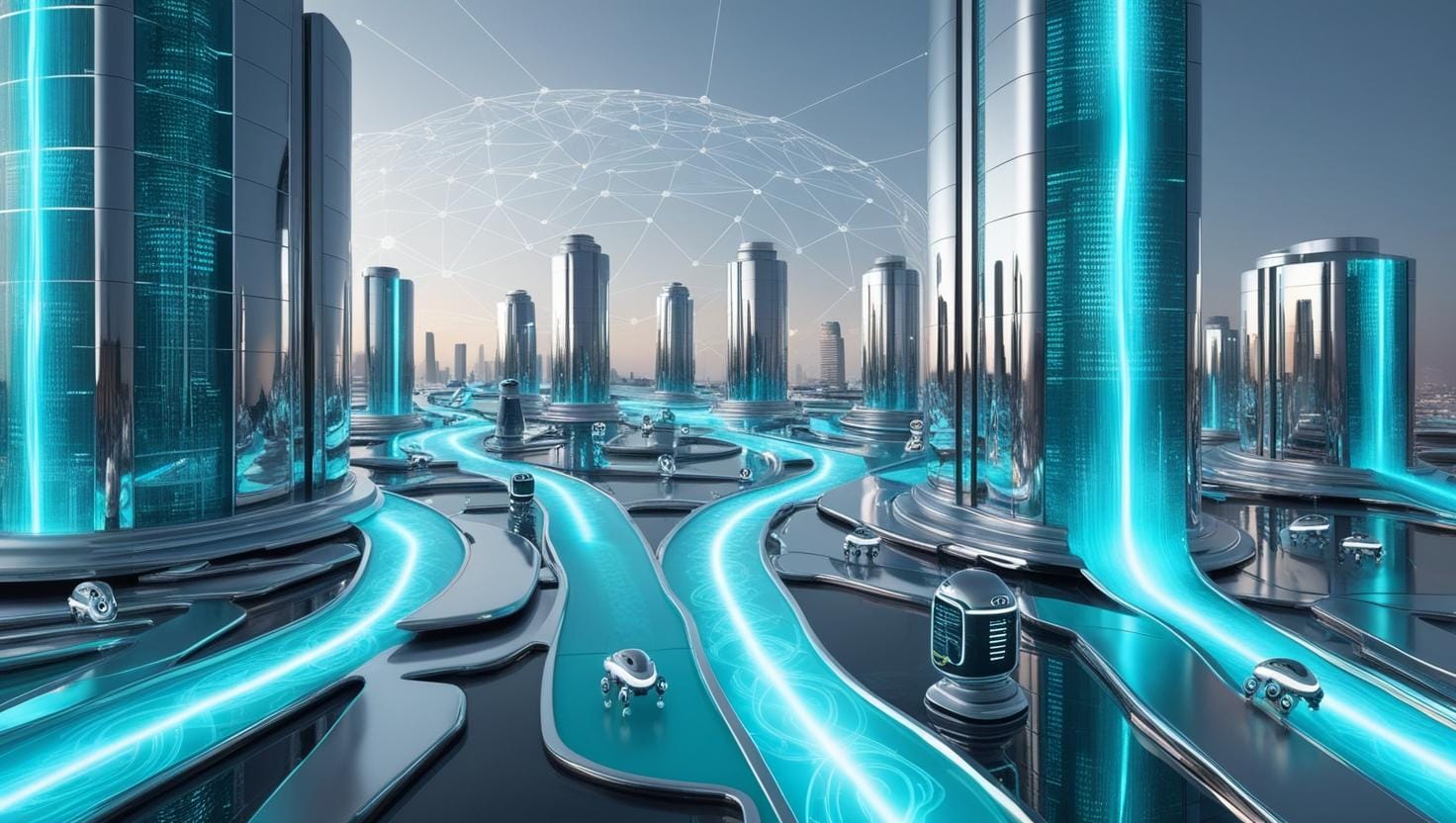
Why a Data Strategy is Mandatory for Success (with AI or not)
In today’s rapidly evolving technological landscape, data strategy has transitioned from an optional consideration to a fundamental prerequisite for any organization aiming to implement artificial intelligence (AI) effectively. Companies that overlook the importance of a well-crafted data strategy risk stalling their AI initiatives and losing competitive ground in a data-driven economy.
In today’s landscape, a company that lacks a data strategy resembles a ship adrift without a compass, lost in the competitive market. Data serves as the currency of the modern digital economy, and organizations that fail to manage it strategically find themselves at a disadvantage against those that leverage its power. When looking to implement AI's wide-ranging benefits—from predictive analytics to process automation—the lack of a solid data strategy is clear: these firms will fall behind. Without a strong data foundation, AI transforms from a revolutionary tool into an unreachable goal. Furthermore, without a defined data strategy, companies become oblivious to market changes, which hinders their ability to respond to shifts and reduces their agility in adapting to new trends and consumer needs.
Here’s why every company, regardless of size or industry, must prioritize building a robust data strategy—and how this strategy serves as the backbone for AI-driven transformation.
What is a Data Strategy?
A data strategy is a comprehensive and understandable plan that outlines how an organization collects, manages, processes, and uses data to achieve business objectives. It is the foundation for aligning data initiatives with broader company goals, ensuring that data is treated as a strategic asset rather than an operational afterthought. In essence, a robust data strategy empowers organizations to unlock the full potential of their data, transforming it into actionable insights and competitive advantages.
Until recently, data strategies were primarily focused on supporting analytics, business intelligence (BI), and reporting functions. The goal was clear: provide decision-makers with the tools and insights to monitor performance and make informed choices. However, the emergence and rapid advancement of artificial intelligence (AI) have dramatically altered the role of data strategy. Today, data strategy has evolved from being a support function to becoming the core of any forward-thinking organization.
With AI on the horizon, data is no longer just a byproduct of business operations but the lifeblood of AI-driven innovation. A well-crafted data strategy ensures organizations have high-quality, structured, and reliable data for training and deploying AI systems. It also establishes governance, security, and ethical use frameworks—key components for maintaining trust and compliance in an increasingly regulated digital landscape.
Key Shifts in Data StrategyI’m pointing out these three shifts:
- From Supporting to Leading: Historically, data strategies supported operational functions like reporting and BI. Now, they lead transformations by enabling AI, predictive analytics, and automation at scale.
- AI-Driven Objectives: Modern data strategies ensure data readiness for machine learning and AI, emphasizing quality, governance, and accessibility.
- Integration with Business Strategy: Data strategies are no longer siloed. They fully integrate with overall business strategies, influencing product development, customer experience, and market adaptation.
By embracing the principles outlined in some frameworks and adapting them to AI's demands, organizations can ensure that their data strategies remain at the forefront of innovation, enabling them to thrive in an era of rapid technological change.
Key Components of a Data Strategy and Their Roles & Responsibilities
A robust data strategy is essential for organizations to manage, govern, and leverage their data effectively. Below are the key components of a data strategy and the roles and responsibilities of various stakeholders involved.
1. Data Collection
Objective: Establish systematic and transparent processes to gather relevant data from diverse touchpoints, including data from internal systems, external sources, sensors, and user interactions.
Key Responsibilities:
- Data Engineers: Design and maintain data pipelines for data ingestion, integration, and processing from various sources.
- Business Analysts: Identify key data requirements, ensuring alignment with business objectives and strategies.
- IT Teams: Oversee the infrastructure needed for data collection, including APIs, databases, and cloud services.
- Data Owners: Define and validate the sources of truth for critical datasets, ensuring they are reliable and relevant.
2. Data Governance
Objective: Define clear ownership, compliance frameworks, and security measures to ensure the organization's ethical and secure use of data.
Key Responsibilities:
- Data Governance Leads: Establish and enforce data policies, standards, and procedures to maintain data quality, security, and compliance.
- Legal and Compliance Teams: These teams ensure adherence to regulations and industry standards governing data privacy and usage, such as GDPR, CCPA, and HIPAA.
- CISO (Chief Information Security Officer): This role protects data against breaches, unauthorized access, and cyber threats by implementing robust security measures and access controls.
- Stakeholders Across Departments: Participate in governance committees to align on best practices, monitor compliance, and foster a culture of data responsibility.
3. Data Quality
Objective: Ensure data accuracy, consistency, and reliability through validation procedures and ongoing assessments to ensure data is fit for use.
Key Responsibilities:
- Data Quality Analysts: Monitor data integrity, conduct regular audits, and resolve inconsistencies or inaccuracies.
- Data Stewards: Maintain and enforce quality standards for datasets within their domain, ensuring data meets predefined criteria for accuracy and consistency.
- Data Scientists: Provide feedback on data usability for analytics, models, and AI initiatives, ensuring high-quality input for advanced analyses.
- Business Units: Validate that data meets their operational requirements, ensuring it supports decision-making and drives outcomes.
4. Data Integration
Objective: Seamlessly align the data strategy with the organization’s digital transformation initiatives, integrating disparate systems and enabling synergy across data sources.
Key Responsibilities:
- Integration Specialists use appropriate integration tools to ensure the smooth interoperability of data systems across various platforms, applications, and databases.
- IT Teams: Manage ETL (Extract, Transform, Load) processes and data flows and maintain seamless integration between internal and external data systems.
- Product Teams: Align data integration efforts with application development, ensuring data structures support product features and user needs.
- Executive Leadership: Set strategic priorities for data integration, ensuring alignment with business goals and technology roadmaps.
5. Data Utilization
Objective: Leverage data to drive actionable insights, improve decision-making, and fuel innovation across the business.
Key Responsibilities:
- Data Analysts: Analyze data, extract meaningful insights, and communicate findings to stakeholders for strategic decision-making.
- AI/ML Engineers: Build and optimize machine learning models using curated datasets, enabling data-driven predictions, automation, and innovations.
- Marketing and Sales Teams should use data to personalize customer engagement, refine marketing strategies, and improve sales forecasting.
- Operations Teams: Apply data insights to enhance efficiency, improve performance, and streamline processes.
6. Data Lineage
Objective: Establish a clear understanding of how data flows, is transformed, and is utilized across the organization, ensuring transparency and traceability of data through its lifecycle.
Key Responsibilities:
- Data Lineage Specialists: Implement tools and processes to track and document data flow from source to destination, ensuring visibility and traceability.
- Data Engineers: Map data workflows, transformations, and storage systems, maintaining documentation of all steps in the data lifecycle.
- Data Governance Leads: Ensure data lineage is incorporated into governance policies, enabling transparency for audits, compliance, and troubleshooting.
- Business Analysts: Use lineage data to validate data sources and ensure the accuracy and reliability of reports and analyses.
- IT Teams: Provide the necessary infrastructure and support to enable data lineage tracking across different systems and tools.
Key Benefits:
- Transparency: Clear visibility of data movement and transformation processes makes identifying issues or discrepancies easier.
- Auditing and Compliance: Enhanced ability to track and report on data usage, ensuring compliance with regulations.
- Data Quality Assurance: Helps identify gaps or weaknesses in data processes, enabling better management and improvement of data quality.
Each of these components plays a vital role in the success of a data strategy. The Data Collection process ensures that the organization gathers relevant data, while Data Governance secures it and ensures compliance. Data Quality ensures the data is reliable and Data Integration flows seamlessly across the enterprise. Data Utilization transforms data into insights, and Data Lineage provides transparency and traceability to ensure trust and compliance. By aligning these components, organizations can unlock the full potential of their data and drive meaningful business outcomes.
Benefits of Role Clarity
Establishing roles and responsibilities ensures accountability, streamlines workflows, and reduces redundancy. It also fosters cross-departmental collaboration, a critical element for the success of any data strategy. By aligning these roles with business objectives, organizations can ensure that their data strategy meets technical requirements and delivers measurable value.
By addressing the “what” and the “who” of data strategy, companies create a cohesive framework bridging the gap between data initiatives and their overarching strategic goals. This dual focus empowers organizations to stay agile, competitive, and innovative in an increasingly data-driven world.
Strategy Alignments
By aligning these components with strategic business objectives, companies can create a cohesive approach that transforms data into a powerful driver of growth and innovation. Each component plays a critical role in enabling not just AI readiness but also overall organizational success.
These components aren't just nice-to-haves for organizations aspiring to implement AI but are essential.
Data Strategy as the Foundation of AI
AI systems heavily rely on the quality, quantity, and relevance of the data they are trained on. Without a strong foundation achieved through a well-implemented data strategy, AI promises such as increased productivity, growth, and innovation will likely remain unfulfilled. Challenges such as incomplete datasets, inconsistent formats, a lack of context, and inadequate data classification can significantly hinder AI initiatives.
When properly aligned with business objectives, a data strategy enables organizations to:
- Build scalable data infrastructures.
- Integrate AI seamlessly into operations.
- Reduce the time to extract actionable insights to inform decision-making.
- Introduce a more significant and more precise overview of the markets.
The Role of Data Quality: A pivotal aspect of any data strategy is ensuring data quality. Companies should implement quality gates, such as automated validation tools and periodic audits, to maintain high standards. This reduces human and system errors and ensures that AI models are fed accurate and reliable information.
Data Maturity Levels
Data maturity refers to how an organization can effectively manage, analyze, and use data to drive business decisions. The following table outlines a typical data maturity model, ranging from early stages (ad hoc) to highly optimized and data-driven organizations.
Explanation of Concepts
- Maturity refers to the degree of sophistication with which an organization handles its data. As organizations move from a basic ad hoc state (Level 1) to a more refined and optimized state (Level 5), they achieve greater control, consistency, and capability in leveraging their data for business value.
- Documentation: Proper documentation is crucial to ensure data is understood, accessible, and reusable. In many organizations, poor documentation hinders the ability to scale data practices, integrate with new systems, and maintain consistency across teams. The lack of documentation often stems from low data maturity, where processes are not formalized, and teams work independently.
- Processes: Standardized processes ensure consistency, efficiency, and alignment with organizational goals. Data maturity levels reflect how well these processes are defined, maintained, and enforced. Companies risk inefficiencies, errors, and misaligned strategies without transparent and standardized data processes, which can significantly affect project outcomes.
Relationship Between Maturity and Project Failure
As organizations increase their data maturity, they typically experience more successful outcomes in their data-driven projects. The table below illustrates how varying levels of data maturity correlate with the likelihood of project failure.
Key Takeaways
- Low maturity (Levels 1-2): Organizations in the early stages of data maturity are prone to project failure due to issues such as data silos, poorly defined processes, and lack of standardized documentation.
- Medium maturity (Level 3): As companies develop a more structured approach to data management, they experience fewer failures, although gaps in integration and documentation can still present challenges.
- High maturity (Levels 4-5): Highly mature organizations have established processes and systems that minimize project failures, using data as a key driver of success. However, ongoing data complexity and documentation management remain crucial even at these levels.
In conclusion, organizations that invest in improving their data maturity significantly reduce the risk of project failure. Data maturity is an essential factor in the success of data-driven initiatives.
Aligning Data Strategy with Company Strategy
A strong data strategy doesn’t operate in isolation; it must support and amplify the organization’s overarching goals. When data initiatives are directly aligned with business objectives, companies can unlock immense value, improve adaptability, and foster innovation. This alignment ensures that every data-related activity—from collection to utilization—contributes to the company’s mission, vision, and strategic outcomes.
How to Align Data Strategy with Company Strategy
- Understand Business Objectives
- Implementation: Start by profoundly understanding the company’s strategic goals, such as entering new markets, improving customer experience, or driving operational efficiency. Conduct workshops with leadership and key stakeholders to define priorities and identify how data can support these objectives.
- Advantage: Ensures that data initiatives are relevant and focused on delivering tangible business results.
- Define Key Performance Indicators (KPIs)
- Implementation: Establish measurable KPIs that bridge business goals and data outcomes. For instance, if the objective is to enhance customer retention, track metrics like churn rate reduction, customer lifetime value, and engagement scores.
- Advantage: Provides a clear framework for measuring the impact of the data strategy on business performance.
- Create a Data-Driven Culture
- Implementation: Promote a culture where data informs decision-making at all levels. This includes training employees, embedding analytics into daily workflows, and fostering a mindset that values data accuracy and ethical usage.
- Advantage: Increases organizational agility and alignment between individual efforts and strategic goals.
- Align Data Projects with Strategic Initiatives
- Implementation: Prioritize data projects that directly support strategic initiatives. For example, if entering a new market is a priority, focus on data collection efforts that provide insights into consumer behavior and competitive analysis.
- Advantage: Maximizes ROI on data investments by ensuring they contribute directly to strategic goals.
- Engage Cross-Functional Teams
- Implementation: Establish cross-departmental data teams that include representatives from business units, IT, and analytics. These teams ensure that data initiatives reflect the organization's needs and align with broader strategies.
- Advantage: Breaks down silos, improves collaboration, and ensures a unified approach to data strategy.
- Leverage Advanced Technologies
- Implementation: Use AI-driven analytics, real-time data platforms, and predictive modeling to connect data insights with business objectives dynamically.
- Advantage: Enhances identifying new opportunities, adapting to market changes, and optimizing operations.
- Continuous Feedback and Refinement
- Implementation: Regularly review data strategy performance against business goals through dashboards and reports. Adjust the strategy based on feedback from stakeholders and evolving market conditions.
- Advantage: Keeps the data strategy relevant and aligned with changing business needs.
Advantages of Aligning Data Strategy with Company Strategy
- Enhanced Decision-Making Capabilities
- Data-driven decisions are faster, more accurate, and more aligned with strategic goals, reducing the risk of missteps.
- Identification of New Revenue Opportunities
- Insights from data can reveal untapped markets, product opportunities, or customer segments.
- Operational Optimization
- Streamlined processes and improved resource allocation reduce costs and enhance efficiency.
- Compliance and Risk Management
- Alignment ensures that regulatory and ethical considerations are integrated into the data strategy, mitigating risks.
- Increased Agility
- A data strategy aligned with company objectives enables quicker responses to market changes and shifts in customer preferences.
- Stronger Competitive Advantage
- Companies that integrate data strategy with their overall strategy gain a deeper understanding of the market and a significant leverage over competitors.
The Future of Data Strategy: Trends and Innovations
The data strategy landscape is rapidly transforming as new technologies and approaches emerge to tackle the challenges of scalability, accessibility, and governance. Staying ahead of these innovations is crucial for organizations to future-proof their data strategies and remain competitive. Here are some key trends and innovations shaping the future of data strategy:
1. Data Mesh Architecture
Data Mesh is a decentralized approach to data architecture that aims to replace traditional monolithic systems, where all data is stored and managed in a central repository. In a Data Mesh, data is distributed across various domains, with each domain team taking ownership of its data.
Key Benefits:
- Scalable and flexible architecture.
- Improved data ownership and domain expertise.
- Reduced bottlenecks in data processing.
This approach is particularly beneficial for organizations that have grown too large for traditional centralized data management and need a more agile and decentralized model.
2. AI-Augmented Data Management
AI and machine learning (ML) transform how companies manage their data. From automating data classification and cataloging to enhancing data governance, AI makes data management more efficient and intelligent.
Key Innovations:
- Automated Data Classification: AI tools help automatically categorize data based on content and context, ensuring more accurate data labeling.
- Smart Data Governance: AI-driven systems enforce compliance and governance policies, detect anomalies, and manage data lineage in real time.
- Predictive Data Management: AI can predict potential data issues (like quality or integration problems) before they impact business operations.
These capabilities streamline data management processes, reduce manual effort, and improve decision-making accuracy.
3. Real-Time Analytics
The increasing demand for real-time insights drives the evolution of data streaming and processing technologies. Real-time analytics empower organizations to make decisions on the fly, enhancing their ability to react swiftly to changes in the market or operations.
Key Trends:
- Event-driven architecture (EDA) allows systems to respond to real-time events, enabling instant data processing and analysis. EDA systems are highly scalable and can react to customer interactions, operational triggers, or market events as they occur.
- Edge Computing: With more data being generated at the edge of networks (e.g., IoT devices), edge computing brings data processing closer to the source, reducing latency and enabling faster decision-making.
- Data Streaming Platforms: Tools like Apache Kafka, Apache Flink, and AWS Kinesis revolutionize how data is ingested and processed in real time. These platforms allow businesses to analyze data streams in near-instantaneous time.
Real-time analytics are crucial for industries like e-commerce, financial services, and manufacturing, where timely insights can lead to competitive advantages.
4. Privacy-First Strategies
As data privacy concerns continue to grow, companies are increasingly adopting privacy-first strategies to ensure compliance with global regulations such as the GDPR (General Data Protection Regulation). These strategies emphasize data collection and usage transparency, control, and accountability.
Key Focus Areas:
- Data Minimization: Collecting only the data necessary for specific purposes.
- Secure Data Sharing: Implementing encryption, anonymization, and safe access controls for sharing sensitive data.
- Privacy by Design: Incorporating privacy considerations into every stage of data management, from collection to storage to analysis.
A strong privacy-first approach ensures compliance and builds customer trust, which is increasingly becoming a competitive differentiator.
5. Event-Driven Architecture (EDA)
Event-driven architecture (EDA) is becoming crucial to modern data strategies. It allows organizations to process events in real-time. EDA focuses on producing, detecting, and reacting to events (such as user actions, system updates, or external signals) as they occur rather than relying on batch processing.
Key Benefits:
- Agility: Enables quick reaction to changing conditions or unexpected events.
- Scalability: Easily scalable to handle large volumes of events in distributed systems.
- Improved Decision-Making: Organizations can base decisions on up-to-the-minute data, making them more responsive and data-driven.
EDAs work particularly well in customer-centric industries, such as retail or entertainment, where the customer experience can be enhanced by reacting to events like purchases, service requests, or interactions with digital platforms.
6. Event Mesh
An Event Mesh is a network of event-driven applications and systems that enables seamless communication between them by allowing events to be sent, received, and processed across a distributed environment. It connects various event-driven systems to ensure data flows efficiently between different parts of an organization.
Key Benefits:
- Increased Interoperability: Facilitates communication across diverse systems (cloud, on-premise, hybrid) without requiring significant re-engineering.
- Real-Time Data Distribution: This feature enables real-time data sharing across departments, locations, or external partners, driving more informed decisions.
- Flexibility: Event Meshes support cloud-native and legacy systems, ensuring they can coexist and communicate effectively.
Organizations looking to maximize their data’s value and streamline operations should consider adopting an Event Mesh as part of their broader data strategy, ensuring real-time event processing and improved collaboration across the enterprise.
7. Data FabricA Data Fabric provides an integrated layer of data management and governance that connects various data sources across multiple environments. It simplifies data discovery, access, and sharing, enabling organizations to manage complex data landscapes more efficiently.
Key Innovations:
- Unified Data Access: A single access point to all data, regardless of where it resides (on-premise, cloud, or hybrid environments).
- Smart Integration: Seamless data integration, even from heterogeneous data sources, without requiring heavy lifting or manual intervention.
- Automated Data Governance ensures compliance and consistency across the data lifecycle by enforcing governance policies using machine learning.
Data Fabric technology is essential for organizations managing vast and complex data landscapes. It enables them to operate with greater agility, security, and scalability.
8. Blockchain for Data Integrity and SecurityBlockchain technology is increasingly being explored to ensure the integrity and security of data. By providing a decentralized, immutable ledger, blockchain guarantees that data cannot be altered without a trace, protecting sensitive or critical business data.
Key Applications:
- Data Provenance: Tracking the origin and history of data to ensure its accuracy and reliability.
- Data Security: Enhancing privacy by allowing only authorized access to sensitive data without needing a centralized authority.
Organizations can enhance trust and transparency by adopting blockchain for data security and integrity, particularly in finance, healthcare, and supply chain management.
Conclusion: Future-Proofing Data Strategy & Why It’s Non-Negotiable
In today’s data-driven world, a robust data strategy is not just an option—organizations must remain competitive, agile, and innovative. As technology advances, key trends such as Data Mesh, AI-augmented management, Event-Driven Architecture, and privacy-first strategies reshape how organizations approach data. These trends ensure data is used efficiently and securely and help businesses stay ahead in an increasingly complex environment.
A strong data strategy provides the foundation for leveraging emerging technologies like AI and real-time analytics, driving improved decision-making, and enabling businesses to uncover deeper insights. Moreover, it ensures that companies are ethically and legally compliant with regulations such as GDPR and CCPA, mitigating the risks associated with data breaches and misuse.
Ultimately, a future-proof data strategy allows businesses to unlock the full potential of their data, driving continuous improvement, innovation, and transformative value. As organizations continue to embrace these technological advancements, their ability to navigate data complexities will determine their long-term success.
Key Takeaways:
- Data strategy is non-negotiable: A comprehensive, well-aligned data strategy is the cornerstone of AI implementation and business success.
- Data quality, governance, and integration are critical. Proper data management ensures reliability, security, and efficiency in leveraging insights.
- Alignment with business objectives ensures long-term impact: Tailoring data strategies to organizational goals is crucial for sustained success.
- Staying updated on trends is vital: Embracing innovations such as Data Mesh, AI-augmented management, and real-time analytics is key to remaining competitive.
Teaser for Further Exploration:
AI is only as good as the data supporting it—but a data strategy must go beyond AI to ensure long-term success. If you're serious about building a resilient, scalable, and future-proof data strategy, check out the FORCE methodology by Manuel Levi. FORCE introduces a structured approach focusing on Foundation, Observation, Resilience, Competence, and Expansion. and creating the base for organizations adopting aligned data strategies and paving the way to AI, by leveraging data as a strategic asset.
Ready to take your business and AI initiatives to the next level? We’ll explore how to design a scalable data strategy tailored to each organization’s unique needs. Stay tuned!