Turning Data Challenges into Wins
Discover one way to transforms fragmented data management into a structured and effective governance strategy
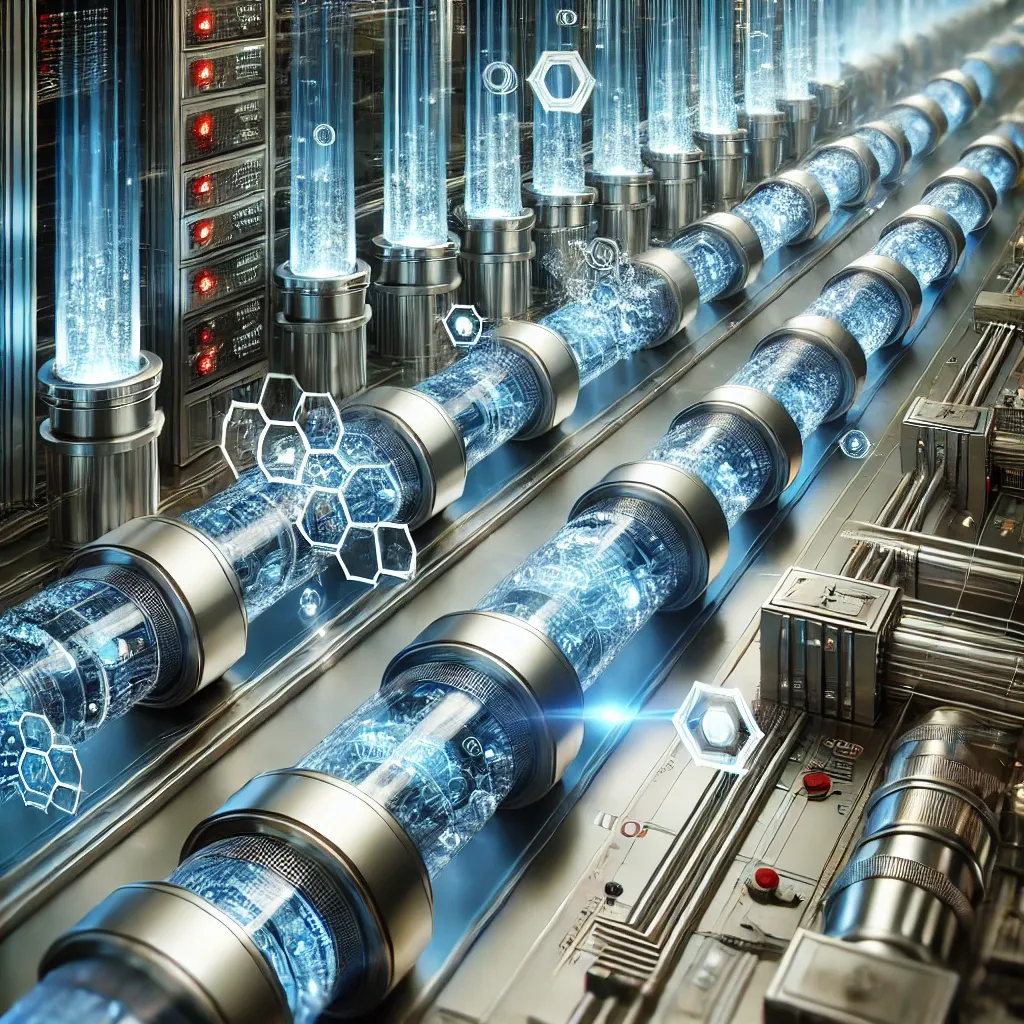
The DRL (Data Readiness Levels): Turning Chaos into Opportunity
The idea of using DRL (Data Readiness Levels) emerged from an engaging conversation sparked by a comment on a recent post about data domains, data silos, and data mesh:
"Interesting insights on Data Mesh and its impact! How have you seen teams successfully switch from data silos to this approach? Would love to hear any best practices or stories."
This question highlighted a common struggle many organizations face, not just breaking down data silos but transforming how data is managed and governed in a way that ensures long-term usability and trust. As the discussion evolved, the need for a framework to classify and improve data readiness became apparent, leading to borrowing some concepts of the DRL framework.
The DRL (Data Readiness Levels): Turning Chaos into Opportunity
The rise of artificial intelligence is pushing companies to take a hard look at their data. Many are discovering a harsh truth: their data is messy, disconnected, and hard to use. Data silos, poor governance, missing lineage, and lack of documentation make it difficult to unlock the actual value of data.
AI thrives on clean, organized, and connected data. However, for most organizations, the gap between their current state and what AI needs feels like a canyon. Fixing this is not easy, but focusing on the correct principles makes it possible to create a more innovative, more connected approach to data.
Here are some key ideas (probably best not to call them best practices) to guide this journey:
Empower Leaders and Teams
To succeed with data, your organization's top leaders must fully support the effort. While the work might begin with technical teams, real change happens when executives prioritize data. Leaders must actively champion the cause, showing everyone that clean, governed, accessible data is non-negotiable.
When leaders support data initiatives and provide the resources needed to achieve goals, they set a clear standard prioritizing data quality, governance, and collaboration across the organization. This top-down emphasis drives accountability and encourages teams at all levels to prioritize these aspects.
Create Strong Rules and Stick to Them
Data governance is not about compromise, it's about consistency. A clear set of rules must define how data is collected, stored, and used. These rules should be strict, and exceptions should be rare. Without a strong foundation, data will continue to be inconsistent and difficult to trust.
Think of governance like building codes. You don't want to live in a house where every room follows different rules; your data systems should be the same. Consistent governance ensures your data "house" stands firm.
Build Tools for Data Visibility
It's impossible to manage what you cannot see. A strong toolchain for data observability is essential. This means setting up systems that track where your data comes from, how it changes over time, and how it's used.
Data observability tools act like a monitoring system for your pipelines and storage. If something goes wrong, you can detect and fix it quickly. These tools also help teams understand how reliable the data is before using it in decisions.
Define and Adjust High-Level Domains
To avoid chaos, organize your data into high-level categories or domains.
These domains give everyone a clear starting point for organizing and governing data. Over time, the domains might need to change as the business grows or processes evolve, but having a structure provides clarity and direction.
Enhance Communication for Data Transformation Visibility
Clear and consistent communication is essential for large-scale data transformation. It ensures everyone is aligned and aware of the progress and challenges in the data journey. Leaders must provide visibility into the transformation process by promoting the channels for sharing updates, insights, and feedback. This creates a trusting environment and allows teams to understand the impact of data initiatives on their work and decision-making.
Just as a strong toolchain is essential for data observability, the communication strategy ensures that information reaches everyone quickly. It drives engagement, promotes collaboration, and keeps everyone in the loop about the organization's data efforts.
Introducing DRL (Data Readiness Levels): A Tagging System for Data Progress
Achieving real value from data requires measuring and improving its quality and governance. Enter DRL (Data Readiness Levels), a framework for tagging datasets based on their current level of governance, organization, and readiness for use.
Like technology readiness levels used in engineering, DRL provides a clear, progressive roadmap for improving data. It helps organizations understand where each dataset stands and the steps to move it to the next level.
Here's how DRL works:
- DRL Levels: Each dataset is assigned a level (e.g., DRL-1, DRL-3, DRL-5) based on how complete and governed it is. Lower levels indicate raw, unorganized data, while higher levels represent entirely governed, lineage-tracked, AI-ready data.
- Certification: Datasets cannot move to a higher DRL without meeting specific quality and governance standards. For example, a dataset at DRL-3 might require basic metadata, while DRL-1 could demand complete lineage tracking, observability, and documentation.
- Communication and Training: The organization needs to understand the DRL framework and how it applies to its work. Leadership must provide clear and visible examples, ensuring that governance is essential not only as a technical requirement but also as a way of being in business, like a culture.
The Goal
The DRL ultimate goal is to unlock your data's potential. When data is well-governed, connected, and reliable, it becomes a powerful tool for decision-making and innovation. AI systems can perform better, teams can work faster, and the organization can move toward its goals confidently.
By following these principles and committing to the DRL framework, your organization can turn its data from a source of frustration into an opportunity.
Footnote:
DRL (Data Readiness Levels) adapts the concept of readiness levels from other industries, providing a structured, measurable way to track and improve data practices. Many organizations have attempted data transformation but have struggled due to unclear milestones. DRL helps solve this by offering a clear progression and criteria for success.